Bringing people together for semantic enrichment
There is a continuing need for the sorts of insights and judgements that only a person can bring, writes David Stuart
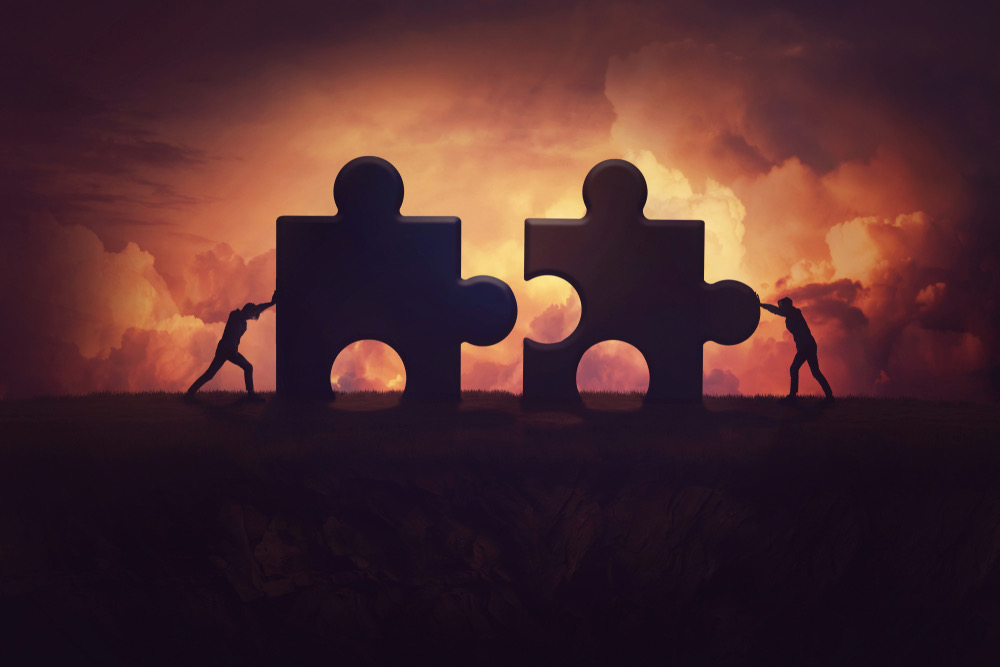
Image: StunningArt/Shutterstock.com
An inconvenient truth of the data age is that so much of the value in the data that we are capturing is just going to waste.
For the value of data to be realised it must be FAIR (Findable, Accessible, Interoperable, and Reusable) and too often it just isn’t. Data is being captured but it’s not in a machine-readable format, or when it is in a machine-readable format it’s not making use of common standards. The waste is unnecessary; solutions are readily available that just need to be implemented.
Semantic enrichment is one such solution that has been around for a while. It tackles the problem of data wastage by reducing the ambiguity in the language that is used. The language that people use every day is naturally rich and ambiguous, containing both synonyms and homonyms, and over time meanings change. This ambiguity often goes unnoticed by people, as there’s generally sufficient context for understanding or the opportunity for clarification. It can quickly cause difficulties, however, when machines are used to find and reuse data. Does mercury refer to the element, the planet, the god, the space program, or one of the countless individuals or organisations that have it as a name?
Semantic enrichment is the process of adding a machine-readable layer of metadata that makes things findable through the disambiguation of concepts with controlled vocabularies. These vocabularies may have different amounts of relational richness; from simple subject headings and authority files, through hierarchical taxonomies and thesauri, to more complex relationships in ontologies and knowledge graphs.
There has been increased interest in semantic enrichment in recent years. Marjorie Hlava, president of Access Innovations, attributes the increased interest to a combination of computing power and greater awareness: 'The biggest change has been the increased power of computers. With cloud computing we have a great deal of power at our fingertips wherever we are, we are able to do a lot of the things people have dreamed about for years but couldn't actually implement.
'The second biggest development is awareness. People are now aware of what semantics can do. Taxonomies and thesauri are very well established and are being widely embraced. Knowledge graphs, knowledge maps, and ontologies are only beginning to be able to be implemented because they're only partially understood, and the search algorithms to actually implement them are few and far between.'
The growth in awareness is particularly important because it allows the emergence of collaborative projects, the building of agreed standards, and encourages additional tools and projects to be built on these standards.
Collaborative solutions
Pistoia Alliance’s SEED project is one such collaborative semantic enrichment project. The Pistoia Alliance is a not-for-profit alliance, with more than 200 member companies and organisations from pharma, biotech, and the life sciences, with a mission to lower boundaries in Research & Development, and to encourage innovation. As Gabrielle Whittick, the lead on the SEED project, noted: 'Semantic enrichment is a perfect example of how we can help with that, we are able to collaborate across pharma, across different organisations.'
The SEED Project focuses on incorporating an additional layer of semantic data into electronic lab notebooks (ELNs), as Whittick continued: 'In R&D in science today the data volumes are increasing exponentially, and we're not able to get the value out of that data that we should be. There's an incredibly high percentage of data captured in R&D that is not really useable.'
'If we can enrich the unstructured text that the scientist is capturing, which is the record of the experiment, then you have information that you can search on, very powerfully. You can analyse it and make decisions based on that information, because you know it's consistent, it's high quality. It's information that you can share externally and internally and it's going to be the same information because we've used the same standards.'
Collaboration between organisations is an important part of the development of these standards, not only to ensure the widest possible knowledge acquisition and efficiencies in production, but because the standards are more valuable the more widely they are adopted.
Whittick explained: 'The success of these types of activities is purely dependent on collaboration across different competitors, and that collaboration only comes with building trust and therefore encouraging sharing.
'Pistoia allows that to happen. It is quite unique in a way, with its legal framework for pre-competitive collaboration bringing together a cross industry project team, and it takes quite a while to build up that trust. But once you have done it, and the sharing begins, it's like a eureka moment. You can make so much of a difference together. The value of that is just huge. Semantic enrichment was initially brought to Pistoia as an idea by Pfizer. They were already doing semantic enrichment, but they recognised it would be fantastic if this was done across pharma, and if we worked together on this problem, rather than trying to solve it individually.'
The project has focused on the development of new assay ontologies for ADME, PD, and drug safety. The first phase worked on ensuring everyone was using the same terms, and then the second phase built relationship maps on those terms that had been agreed upon and have been published in the BioAssay Ontology. The project then developed an exemplar which showed what it would look like as part of an ELN workflow: starting with an unstructured text, using semantic enrichment and an API to connect with the standards that are used across the industry, and leaving at the end an enriched text. The next is looking at breadth rather than depth, working on the data model for an experiment.
Companies are already reporting the realisation of value from the project – and, as Whittick explained: 'Any company who thinks they are going to do it on their own is very short sighted. They can't reap the same value doing it on their own. They are spending resources trying to solve a problem that they don't need to, because we're solving it together.'
Drawing on different perspectives
While collaboration within an industry is important for developing, sharing, and building standards, in other situations vocabularies will want to include as wide a range of perspectives as possible. This can be an important part of making things more equitable, ensuring different groups and points of view are represented, and their associated data and documents are findable. While this is increasingly recognised for many historical collections, it can nonetheless be a divisive issue when it comes to subjects that cross political divides.
As Hlava pointed out, choices in terminology can lead to semantic censorship. While it may not be a conscious choice, in can lead to a bias in the terms that are taken and the directions subsequent analysis takes: 'Semantic censorship is pervasive, wherein we want to make sure that whatever term is applied could be used by all the communities that generate or need to find or discover information. For example, when the Covid pandemic hit I decided it would be really helpful and a good service to our clients, and anybody who wanted it, to build a taxonomy of Covid terms, and I very quickly came up with about 20 synonyms for what we now generally call Covid-19, as well as related terms for drugs and treatments.
'I presented the list to one of my editorial staff, and the list included ‘Wuhan Virus’ and ‘CCP virus’, and he exploded, and said you can't include those terms in the thesaurus because they are derogatory. But if we don't include them in the thesaurus we are missing all kinds of publications and discussions that would probably be necessary for researchers to read. We want them to get all that information. We have to be inclusive. Our job is not to decide which is the proper term, or which group has the right information. Our job is to record it all.
'It leads to the whole question of equitable discovery, because if group A holds one opinion and group B hold another and group C holds yet another point of view, we want to be sure that all those parties are working from the same body of information. We can't decide what their conclusions are going to be, but you want people to have all the information available. I don't care what decision they make, that's not my job. My job is to make sure they have all the information. It can be accumulated on the topic and then they can makeup their own mind.'
The difficulties can grow exponentially when working on multilingual taxonomies, or trying to align concepts from differing world views but, as Hlava explained, work can potentially have far reaching consequences in the political sphere: 'We aren't usually aware of those mappings, but I frequently think that part of the reason we don't quite understand our enemies is we don't really get inside of their outlines of knowledge, their knowledge organisation for the country and for the school of thought they follow as opposed to our own. That causes a lot of unnecessary misunderstandings, we are coming at the problem from another direction of thought.'
The need for semantic enrichment
While there is an increased recognition of the need for semantic enrichment, it is not the only potential tool being promoted as a solution to the data wastage problem. Artificial intelligence is often seen as the exciting solution. As Whittick pointed out, however, It’s not a case of one or the other, but often a case of one building upon the other: 'We need to build standards, and no one’s very excited about that in many ways, but unless we have those building blocks, you can't really go ahead with your AI and machine learning.'
As Hlava explained, the failure to understand the role of semantic enrichment is often coupled with a popular misunderstanding of what artificial intelligence means, and the amount of guidance the technology still needs: 'Part of the challenge is that artificial intelligence is a bit of a misnomer. A lot of what people think of as artificial intelligence are algorithms which automate a repetitive process that humans do. Automating a repetitive process is very well advanced, and very sensible, but to me that's still not artificial intelligence.
'Artificial intelligence is where people add the machine learning algorithms, and machine learning learns and improves the algorithms consistently through all kinds of vectors and statistics, neural maps, and lots of other techniques. But the machine keeps on learning, and unless people keep looking at it they don't have a chance to augment the algorithms, and the machine keeps on going in a straight trajectory and it can learn the wrong things. It might learn things we don't think are morally or intellectually quite appropriate. It becomes a little dangerous if we let them run unsupervised.'
Artificial intelligence doesn’t offer a panacea to our data wastage, rather it should be recognised as a tool that can run in harmony with semantic enrichment rather than in competition. There is a continuing need for the sorts of insights and judgements that only a person can bring.
Semantic enrichment is an important part of tackling many of the big problems facing the world today. The sorts of problems that are encapsulated in the UN’s Sustainable Development Goals requires us to start making far better use of the data that is already available, whether we are talking about tackling scientific or social and political problems, whether we are capturing data in the lab or perspectives across divisive issues.
For semantic enrichment to be most beneficial, however, it must be collaborative and inclusive.